
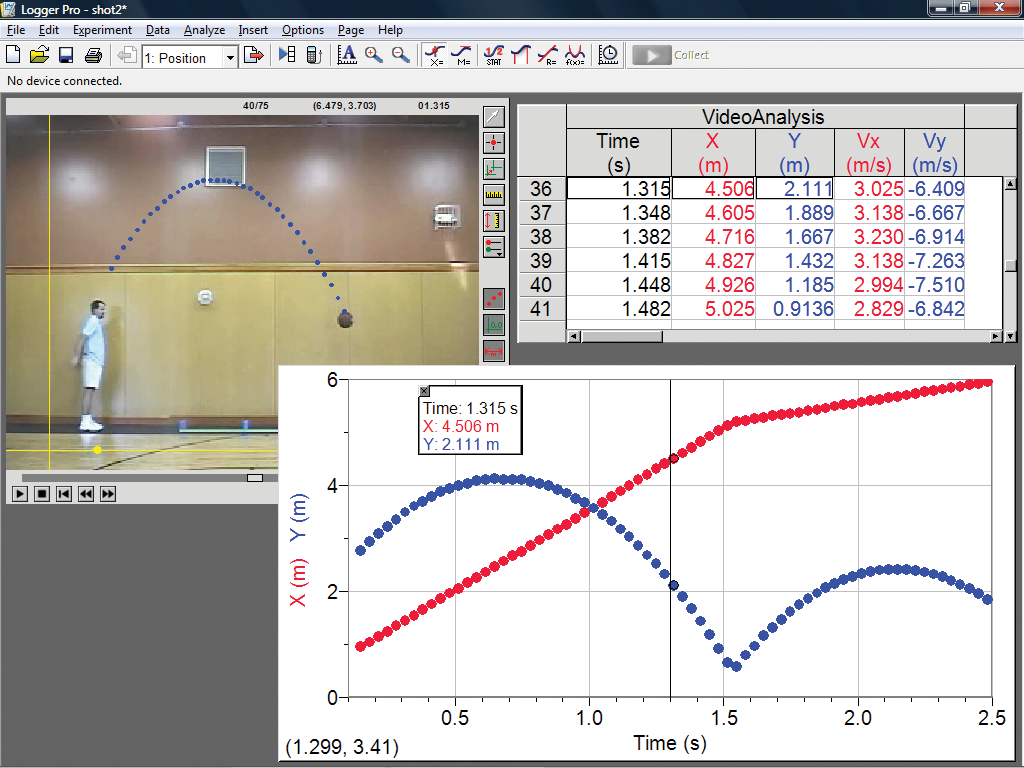
The Kalman filter is a recursive state estimator of a discrete-time controlled process governed by a linear stochastic differential equation. The techniques based on variants of the Kalman filter and Sequential Monte Carlo (SMC) method are the most popular tracking and localization methods, respectively. Tracking and localization methods aim to fuse uncertain position measurements to estimate the variable of interest with different assumptions about the representation of the vehicle’s location. The reliability of such a perception is a key for tracking and location purposes. Therefore, the modeling, processing, and fusing of underwater sensor readings are of paramount importance to build a reliable representation of the submarine environment. We should bear in mind that optical and sonar sensors provide a short range of high-resolution uncertain sensing readings, mainly due to the different factors affecting their uncertainty, such as multipath reflections and poor underwater visibility. The effectiveness of localization based on an a priori known representation of the environment depends on the wealth of useful information. A significant advantage of this approach is the cheap cost with a minimum modification of the workplace. We can also use optical or sonar sensors to identify specific landmarks in the environment and use them to locate the underwater vehicle using an a priori representation of the environment.
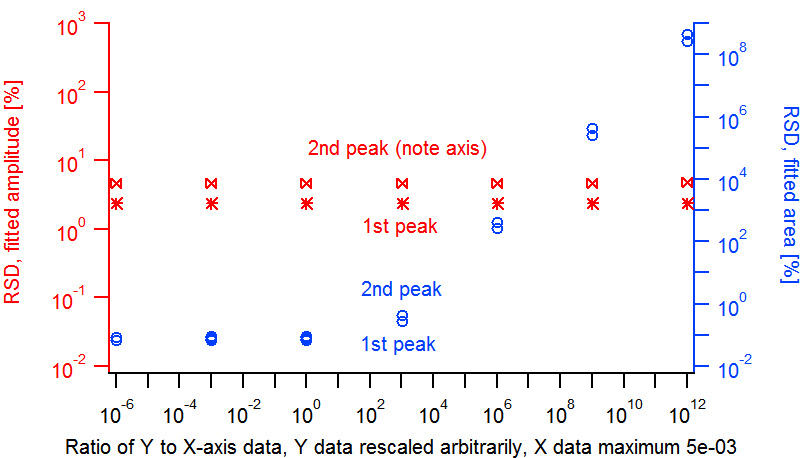
These underwater localization systems also require the deployment of a network of sea-floor mounted baseline transponders, often in the perimeter of the workplace area, for LBL or a support vessel with the transponders following the vehicle for SBL and USBL. However, these sensors suffer from multipath Doppler effects and thermoclines, which induce acoustic reflection effects. Īcoustic positioning systems are the most used underwater external positioning approaches, from long baseline (LBL) to sort baseline (SBL) and ultrashort baseline (USBL). For this reason, several works in the literature combine the information of proprioceptive sensors to external positioning systems. This fact makes unfeasible underwater dead-reckoning navigation. However, these proprioceptive sensors suffer from drift and biases, leading to growing position uncertainty as the vehicle navigates. This is because the submerged vehicle cannot detect the electromagnetic signals provided by the global navigation satellite system (GNSS). Underwater vehicles incorporating a certain degree of autonomy usually rely on proprioceptive sensors, such as an inertial navigation system (INS) integrated with Doppler velocity logs (DVLs). These vehicles can perform many tasks in a broad spectrum of applications, such as inspection, repair, and maintenance in defense, oil and gas, and cable surveying, to name but a few. Nowadays, underwater vehicles allow us access to restricted areas and traditionally dangerous environments for human divers, such as deep seabed and under the ice. Besides, they evaluate the improvement of localization compared to the position estimation using reliable dead-reckoning systems. The experimental results show that the framework provides a reliable environment representation during the underwater navigation to the localization system in real-world scenarios.

We locate the underwater vehicle using a Sequential Monte Carlo (SMC) method initialized from the GPS location obtained on the surface.

We evaluate the framework using cameras and range sensors for modeling uncertain features that represent the environment around the vehicle. By adopting uncertain modeling and multi-sensor fusion techniques, the framework can maintain a coherent representation of the environment, filtering outliers, inconsistencies in sequential observations, and useless information for positioning purposes. Submerged sensory information is often affected by diverse sources of uncertainty that can deteriorate the positioning and tracking. You may assume the uncertainty in position is negligible.This paper presents a framework for processing, modeling, and fusing underwater sensor signals to provide a reliable perception for underwater localization in structured environments. The data set "calibration.txt" shows the _reported_ position of a rotational sensor (in units of $\frac \cos(\omega t + \phi) + \varphi $$įind the resonant frequency $\omega$ and the damping constant $\beta$ for this apparatus.
